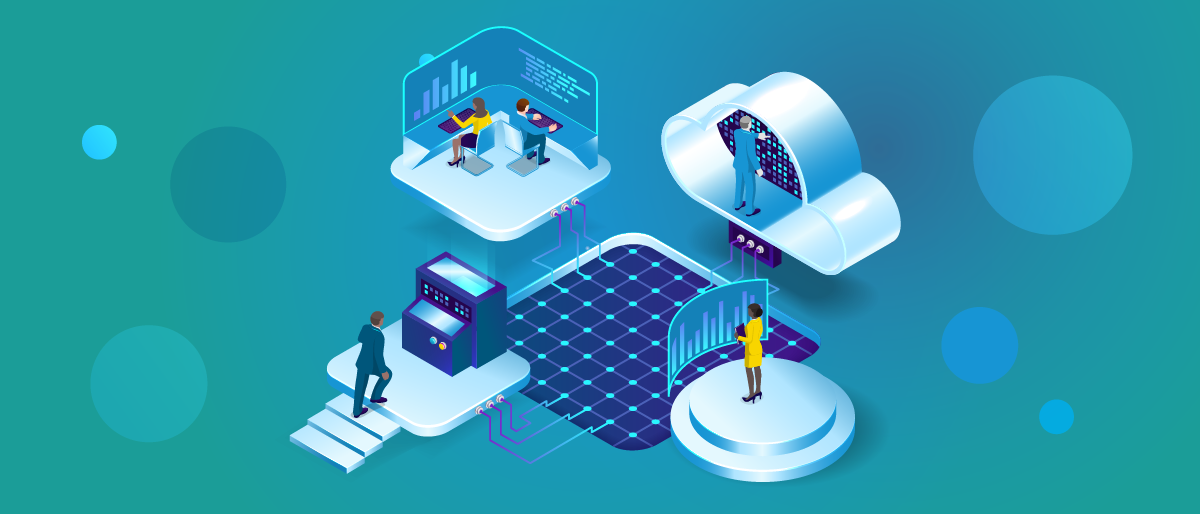
Find out what data scientists answered when asked the question, “What do you see as the main challenges with machine learning?”
July 13, 2022Find out what data scientists answered when asked the question, “What do you see as the main challenges with machine learning?”
July 13, 2022