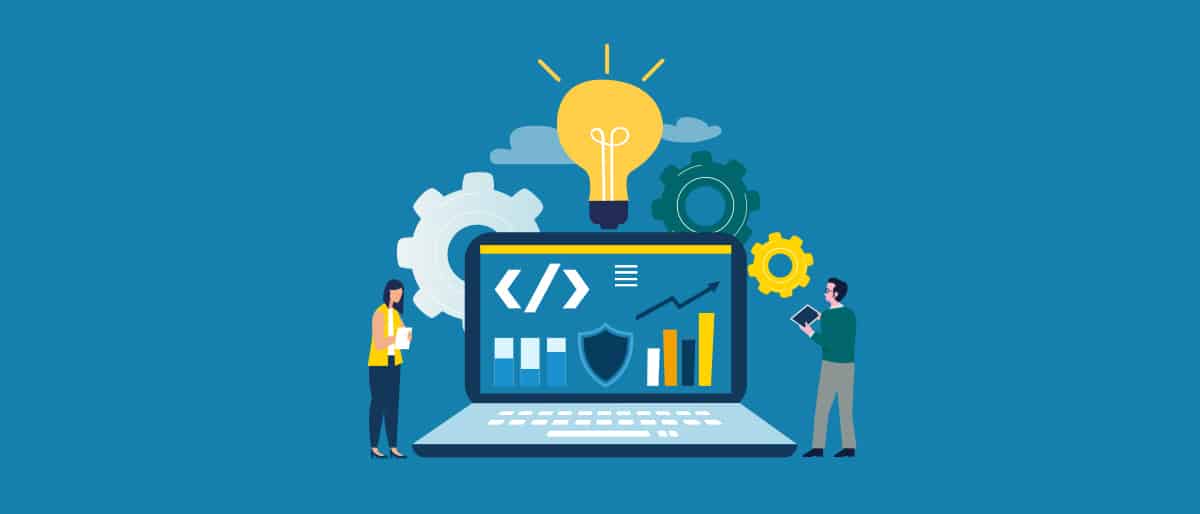
Can product based thinking help make data governance easier? Equal Experts’ Mohit Chandna shares how his product experience helped the implementation of a data catalogue more successful.
March 30, 2023Can product based thinking help make data governance easier? Equal Experts’ Mohit Chandna shares how his product experience helped the implementation of a data catalogue more successful.
March 30, 2023